Many of us wonder how the application knows that a given product – film, program, musical band – will hit our tastes. It is possible thanks to AI, which has analyzed our past behaviors and learned the correct conclusions.
AUTHOR: Michał Marosz, IMGW-PIB/Center of Research and Development
Artificial Intelligence is a set of specific tools that allow machines to make their own decisions. The vision of AI as a threat to humans has become established in pop culture. In reality, AI does not intend to replace people. On the contrary, it is supposed to help us solve complex issues quickly – also those related to weather and climate.
How does it know?
Artificial Intelligence (AI) is a set of methods, algorithms, and machines that allow computers or devices to imitate to some extent human intelligence. An important feature of AI is the ability to improve via the learning process. Each of us deals with artificial intelligence algorithms in everyday situations. Virtual assistants, such as Google Assistant or Siri, are algorithms that enable face recognition and tell us „what to choose.” Artificial Intelligence processes large amounts of data quickly and efficiently, allowing it to identify and quantify patterns that are „hidden” in the data and prepare a proper answer to correctly constructed questions.
Artificial Intelligence more effective in forecasting atmospheric phenomena?
The rapid development of AI over the last decade, especially in Deep Learning, has confirmed the possibility of using such tools in tasks based on non-linear pattern recognition. Atmospheric science has multiple problems that require this approach – weather forecasting is basically a complex forecast of non-linear processes in three dimensions. So the question is whether forecasting models could use methods from the AI tools range?
Machine Learning is a part of artificial intelligence or computational intelligence. It deals with algorithms for analyzing data and learning based on these data. Subsequently, it uses the acquired knowledge, e.g., to predict something. There are four main types of machine learning: supervised, semi-supervised, unsupervised, and reinforced. Deep Learning is a subset of machine learning that deals with classifying, recognizing, detecting, and describing data using deep artificial neural networks – at a deeper level than traditional machine learning.
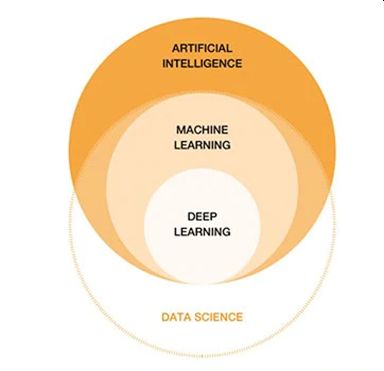
The ability to forecast meteorological phenomena in real-time is one of the essential tasks faced by hydrological and meteorological services today, particularly in the context of extreme events: storms, hail, flash floods, or heat waves. These phenomena’ physics is known and described with some simplicity by dynamic models used in meteorological forecasting. However, it should be stressed that these models have limitations resulting from the characteristics of the applied process parameterization schemes on a subgrid-scale and the availability of computational resources.
Artificial Intelligence – real forecast
The procedure for preparing a weather forecast is complicated. It is a truism, but few people are aware of the actual level of this complexity. The amount of data that has to be processed is enormous: tens of thousands of ground-based observation points administered by the national hydrological and meteorological services, air soundings, satellite images, buoys located in the oceans. The procedure of assimilating data from various sources, combined with quality control and then implementing them into numerical models, is a great challenge. Suppose we realize that it should occur almost in real-time in the case of forecasting extreme violent phenomena. In that case, AI class tools become a potentially indispensable component of the arsenal of hydrological and meteorological services worldwide (e.g., NOAA already uses Artificial Intelligence). Nowadays, the so-called nowcasting (short-term forecasts with a time horizon of up to 6 hours) is primarily based on modelling tools derived from statistical modelling methods, including AI.
Machine Learning or Deep Learning?
Machine Learning (ML) has typically been used in the post-processing of results from numerical models until now. In recent years, the first hybrid models have emerged, e.g., utilized in the parameterization of cloudiness or radiation. The basic assumption of these attempts was to replace numerical models (computationally complex or characterized by significant uncertainty of components) with Machine Learning algorithms, leaving the other components of the model unchanged. This type of approach has many disadvantages – including interactions between numerical components and ML which are not fully recognized. Another problem is the implementation of ML in models primarily written in the Fortran language.
Deep Learning (DL), which uses neural networks with multiple hidden layers, is based on the ability of neural networks to automated pattern identification in multidimensional space described by data. The field of application of DL is vast. In the review article „WeatherBench: A benchmark dataset for data-driven weather forecasting” (https://raspstephan.github.io/blog/weatherbench/), the authors indicate the potential of using Deep Learning in weather forecasting issues.
Potential
Among the issues of AI used in meteorology and climatology, two specific areas can be highlighted: classification and prediction. Most of the analyzed geophysical processes are non-linear processes. The possibility of their correct categorization (in practical use) escapes the most straightforward methods, which do not allow for an adequate analysis of phenomena. An example is the classification of atmospheric circulation processes, primarily responsible for forming weather conditions in mid-latitudes and the analyzed area’s climatic features in the longer term.
Synoptic climatology focused on quantifying relations between atmospheric circulation types (usually at synoptic scale) and environmental conditions usually relies on a specific typology. In the most general outline, there were two approaches: manual typologies (e.g., Osuchowska Klein, Grosswetterlagen, Vangenheim Girs macro forms of circulation) or objective typologies, that are more often referred to as computer-assisted nowadays (e.g., the Lityński typology or generic typology of Ustrnul used in IMGW-PIB).
In each of these procedures – even those called objective a few years ago – at some stage, the researcher is forced to make arbitrary decisions, e.g., regarding the number of defined types. All of this causes that the analyzed image is highly generalized, which does not fully reflect the synoptic situation that resulted, for example, in the occurrence of an extreme phenomenon. The problems of „classical” classification algorithms can be mostly solved using the AI methods catalogue tools.
In the case of classification issues, artificial neural networks, specifically Kohonen’s algorithm, allow for the definition of geophysical field patterns. For atmospheric circulation-related analyses, it is most often the SLP value or the height of a specific isobaric surface. These patterns are classified in the so-called topological map, where similar circulation types are located closer to each other. The problem of determining the optimal number of patterns still exists. However, objective network quality measures allow for an informed selection of the optimal number of types, not dictated by the researcher’s hunch or by performing a significant number of tests in trials and errors mode. This type of analysis was often used in extreme precipitation studies in Japan[1] and South Africa[2], anomalous temperature field characteristics in China[3], and the Atlantic-European region. For example, the variability of atmospheric circulation characteristics was analyzed throughout the 20th century[4].
Czernecki and his team[5] used the Random Forest algorithm’s capabilities to model a large hail (>2 cm) in Poland. The analysis included the use of several dozen parameters describing the state of the atmosphere layer. It allowed not only to develop satisfactory models but also to identify potential predictive variables that could improve the quality of now-casting forecasts of large hail.
Rasp and others[6] attempted to use AI for medium-range forecasts by testing its merits for the 3-5 day horizon. The authors emphasize that the verifiability of contemporary Numerical Weather Prediction (NWP) models is good but requires significant computational resources. This is particularly important when preparing probabilistic ensemble forecasts, where the models repeatedly (several dozen times) generate the course of future meteorological conditions. Models using Machine Learning can be much less demanding in this area. They can generate more ensemble forecast members and improve the overall parameter estimation, which is particularly significant in extreme phenomena forecasting.
Application of AI tools is also possible in the air pollution field, where the set of factors with significant influence on the pollutant’s concentration is considerable, and the application of dispersion models is not possible. Artificial Neural Networks or random forest algorithms allow quantification of non-linear relations between predictors and concentrations of analyzed pollutants. The example can be research led by Jedruszkiewicz, Czernecki and Marosz[7]. The authors examined the possibility of application of varying ML algorithms in the PM concentration forecasts in selected polish agglomerations (Kraków, Łódź, Trójmiasto, Poznań). Nidzgórska-Lencewicz applied artificial neural networks in the analysis on PM concentrations in Tricity Agglomeration[8]. In Silesia Highland Agglomeration many years ago, IMGW-PIB[9].
AI will support us
As you can see, the spectrum of potential AI applications in the analyzes and operational tasks of hydrological and meteorological services is vast. You can risk a hypothesis that with the development of these methods, it will only increase. Nevertheless, doubts are raised as to the interpretability of AI algorithms. Most of them are so-called „black boxes.” Recently, advanced research has been carried out to try to „disenchant” AI in this aspect. Another fundamental question is whether DL models or AI, in general, can „learn” the physics of the atmosphere. This question remains open, although the first results are promising.
In my opinion, weather forecasting will continue to be based on dynamic models in the coming years, the quality of which (verifiability, spatial and temporal resolution, improved parameterization schemes) is constantly evolving. However, it is almost certain that AI methods will have an increasing share in the range of tools used in Earth sciences, especially in meteorology and climatology.
[1] https://doi.org/10.1175/JHM-D-14-0124.1
[2] https://doi.org/10.1007/s00382-015-2836-2
[3] https://doi.org/10.1016/j.atmosres.2019.01.005
[4] https://www.researchgate.net/publication/311777819_Variability_of_atmospheric_circulation_in_Euro-Atlantic_region_1900-2012
[5] https://doi.org/10.1016/j.atmosres.2019.05.010
[6] https://raspstephan.github.io/blog/weatherbench/
[7] https://meetingorganizer.copernicus.org/EMS2018/EMS2018-309.pdf
[8] https://doi.org/10.3390/atmos9060203
[9] https://doi.org/10.1007/978-3-662-04956-3_59